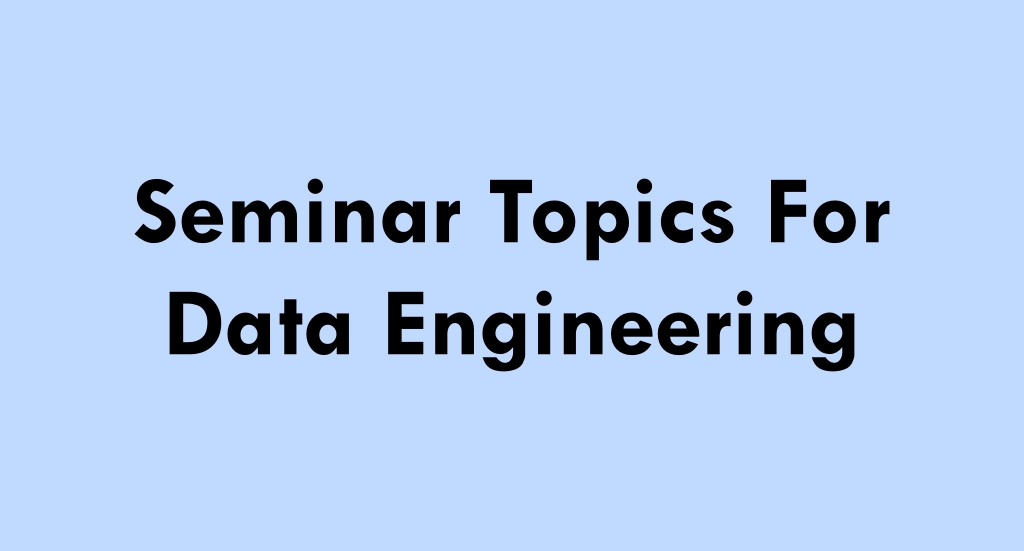
Seminar Topics For Data Engineering | A data engineer develops, designs, constructs, maintains, and manages data architectures, infrastructures, and systems that support collecting, storing, processing, analyzing, and visualizing large amounts of data. Data engineering is a branch of computer engineering.
Seminar Topics For Embedded Systems
Seminar Topics For Mechanical Engineering
Seminar Topics For Data Engineering
Data pipelines: Design and implementation
Real-time streaming data processing using Apache Kafka
Building scalable data processing systems with Hadoop
Distributed computing with Apache Spark
Introduction to Flink for stream processing
Best practices for building data warehouses
Exploring NoSQL databases: MongoDB, Cassandra, and more
ETL automation using Python
SQL vs. NoSQL: Which is right for your project?
Introduction to data warehousing and BI
Building a modern data infrastructure with cloud services
Data modeling for scalable systems
Implementing data governance best practices
Advanced data visualization techniques
Big data and machine learning
The role of data engineering in artificial intelligence
Building scalable data storage systems
Integrating data from multiple sources
Designing and implementing data security measures
Database design and optimization
Exploring data architecture options
Data processing using Apache Nifi
The future of data engineering
The importance of data quality in engineering
Data governance in the age of big data
Data cataloging and discovery
Master data management (MDM) best practices
Data modeling for large-scale systems
How to build a data engineering team
Building data lakes with AWS
Techniques for data integration and transformation
Best practices for cloud data storage and retrieval
Introduction to data streaming
Real-time data processing with Apache Storm
Implementing big data analytics with Apache Hadoop
Understanding the role of data architects
Best practices for data ingestion and preprocessing
Building a data lake with Hadoop and Spark
Designing a data pipeline architecture
Real-time data visualization with Kibana
Implementing a data governance program
Building an end-to-end data processing pipeline
Big data architectures and patterns
Understanding data quality management
Introduction to data governance
Data cataloging and classification
Machine learning for data engineers
Best practices for building scalable data architectures
Cloud data storage and retrieval best practices
Data integration for complex systems
Building a data engineering platform
Best practices for data warehousing
Implementing data governance in cloud environments
Building a big data processing pipeline with Apache Beam
The future of data engineering in the cloud
Techniques for big data processing using Apache Storm
Building a data governance framework
Data modeling and design patterns
Understanding data quality dimensions
Implementing big data analytics using Hadoop and Spark
Data warehousing design patterns
Best practices for data security in cloud environments
Introduction to data virtualization
Building a data pipeline with AWS Glue
Data warehousing with Amazon Redshift
Data governance in multi-cloud environments
Building a scalable data infrastructure with Kubernetes
Techniques for data ingestion and preprocessing in real-time
Understanding big data storage options
Implementing data security measures in big data architectures
Building a data pipeline with Apache Airflow
Advanced data modeling techniques
Implementing machine learning pipelines for big data
Best practices for data privacy
Building a data warehouse with Snowflake
Understanding data lineage
Building a data pipeline with Google Cloud Dataflow
Big data processing with Google Cloud Dataproc
Data governance in hybrid cloud environments
Data Engineering Best Practices for Large-Scale Data Processing
Data Warehousing: From Legacy to Modern Data Engineering Techniques
Understanding Data Integration in Big Data Environment
Data Governance and Quality: Importance in Data Engineering
Data Lineage and Traceability: Challenges and Solutions in Data Engineering
An Overview of Data Lake Architecture and Design
The Role of Apache Kafka in Data Engineering
A Practical Guide to Data Pipelines in Production
Batch vs. Stream Processing: Choosing the Right Data Engineering Approach
The Future of Data Engineering: Trends and Predictions
Big Data ETL Best Practices for Data Engineers
Cloud Data Warehousing: Designing for Scalability and Performance
Data Integration in the Cloud: Challenges and Opportunities
Implementing Data Lakes with Hadoop: Lessons Learned
Data Pipeline Monitoring and Management: Tips and Techniques
Building Robust Data Pipelines with Apache Airflow
Data Security in Data Engineering: Strategies and Best Practices
Data Integration for Machine Learning: Challenges and Opportunities
Designing Data-Driven Applications: From Data Engineering to Data Science
Best Practices for Data Governance in Data Engineering Projects
Building a Data Lake on AWS: Best Practices and Lessons Learned
Introduction to Data Catalogs: Benefits and Challenges for Data Engineers
Creating a Data-Driven Culture: Strategies and Best Practices
The Role of Data Engineering in Business Intelligence
Designing a High-Performance Data Warehouse
The Challenges of Data Cleaning in Data Engineering
Building Data Pipelines with Apache Spark
Understanding NoSQL Databases in Data Engineering
The Role of Data Engineering in Artificial Intelligence
Data Engineering for Real-Time Analytics: Techniques and Best Practices
Designing Data-Driven APIs: From Data Engineering to Application Development
Data Engineering for IoT: Challenges and Opportunities
The Importance of Metadata Management in Data Engineering
Data Profiling in Data Engineering: Techniques and Best Practices
Real-Time Data Processing with Apache Flink
The Challenges of Data Storage in Data Engineering
Data Science Workflows: Designing for Efficiency and Reproducibility
Understanding Data Warehousing Technologies: From Relational to Columnar Databases
Big Data Processing with Apache Beam
The Role of Data Engineering in Data Governance and Compliance
Building a Scalable Data Lake with Apache Hudi
Data Engineering for Data Visualization: Best Practices and Tools
Implementing Streaming Analytics with Apache Kafka and KSQL
The Challenges of Data Consistency in Data Engineering
The Future of Big Data Technologies: Trends and Predictions
Designing Data-Driven Applications with Serverless Architecture
Data Engineering for Natural Language Processing: Challenges and Opportunities
Data Modeling for Data Engineering: Best Practices and Techniques
Designing Data Pipelines with AWS Glue
Data Engineering for Cloud-Native Applications: Best Practices and Challenges
Introduction to Data Engineering for Business Analysts
Data Warehousing with Snowflake: Design and Best Practices
Building Data Pipelines with Talend: Tips and Techniques
Designing a Modern Data Architecture for Enterprise Data Management
Data Engineering for Healthcare: Challenges and Opportunities
Real-Time Analytics with Apache Druid
Data Engineering for Marketing Analytics: Best Practices and Techniques
The Challenges of Data Integration in Multi-Cloud Environments
Designing Data-Driven Applications with Event-Driven Architecture
Data Engineering for Energy Analytics: Challenges and Opportunities